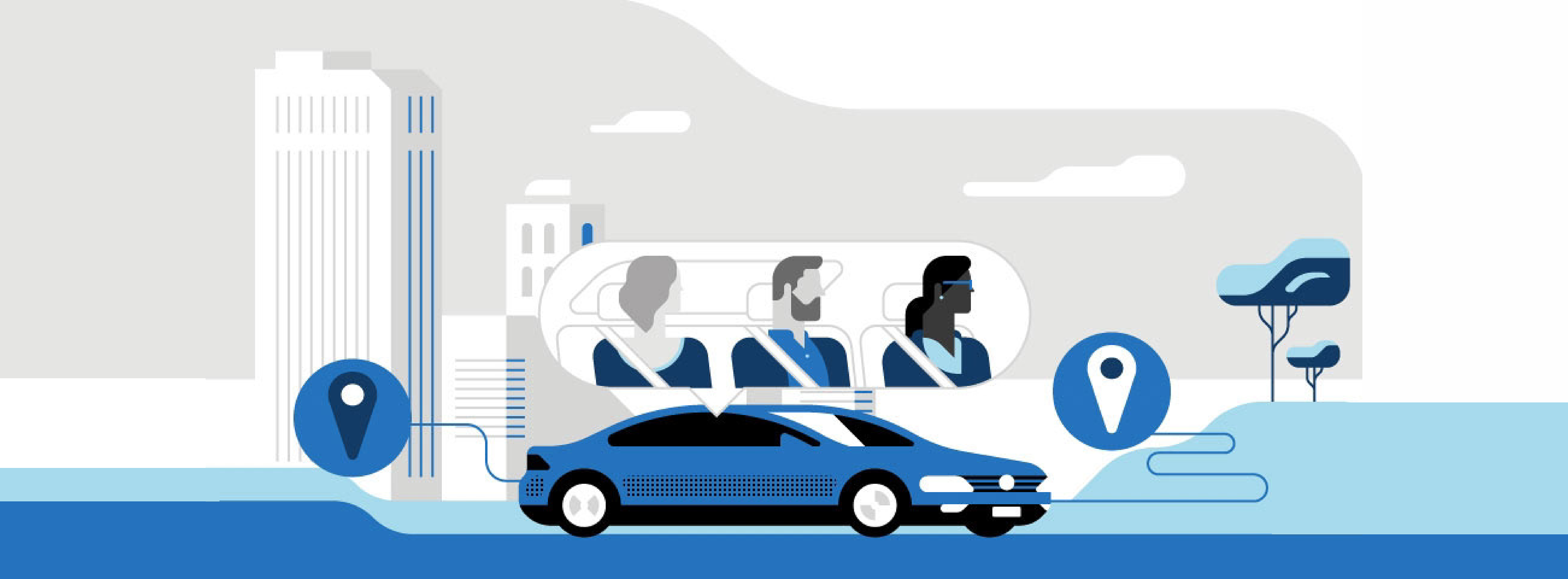
Safety, that’s inherently every person’s natural concern. Humans are hardwired towards safety and protection. Before we get to the statement that “rideshare safety is our responsibility”, let's take a few steps back and look at what’s involved concerning safety. In 2017 “The Future of Life Institute” (FLI), whose founders and board members include Max Tegmark (MIT), Elon Musk, Late, Dr. Steven Hawkins, other key educationists, decision makers from government and futurists held the Beneficial AI 2017 conference’s where they formed the Asilomar AI Principles. Primarily to set of guideline charter for how intelligence, i.e. AI should be beneficial to humans. The 23 guiding principles that were formed are categorized into Research Issues, Ethics and Values, and Long-term Issues. Of the 23 areas in total, #5 which is Race Avoidance - No corner-cutting on safety standards, #6 Safety - AI systems should be safe and secure throughout their operational lifetime, and verifiably so where applicable and feasible .and #22 Recursive Self-Improvement - AI systems designed to recursively self-improve or self-replicate in a manner that could lead to rapidly increasing quality or quantity must be subject to strict safety and control measures, primarily cover “safety”. You may wonder, what is its relevance to “rideshare safety”? Let us pause here for a moment and understand the issues with safety a little deeper from the background perspective. Rideshare has been in existence since the days of wheeled carts. As times and seasons changed progress of man, tools and created intelligence also grew stronger. The concerns of “safety” then were very different then, but mainly towards protection from physical harm, bandits and looting on highways winding down steep hill corners. Rideshare carts were called “stagecoaches” and would even have safety concerns such as falling off the stage, weather and other traveling anomalies. The issues and concerns were because road safety, quality of roads, training of horseman and horses, yes horses were trained to run, walk or behave in a certain manner and that was the job of the horseman. If the horsesmisbehaved it was unsafe and so also if the horseman was drunk or too tired or if his cart was not well serviced, it would pose a hazard or safety issue to its passengers. If there was an ambush en route between towns in the old wild west, that was completely different safety issues which even threatened life. Race ahead in time and we are today in the days of self-driving cars. For ride share one can call for a driverless cab to give us a ride from one place to another. but then the concerns of safety too have changed, no longer do you have to worry about falling off a stage coach or being attacked by gun welding banditos on horseback. It was humans that solved the problems with advancements in technology, tools, improvements in every piece of mobility and mobility as a service has changed over time and with the advancements in technology, primarily on the horizon today is AI.
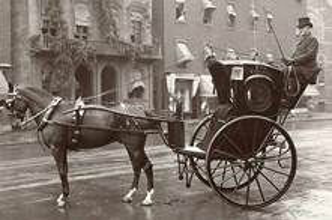
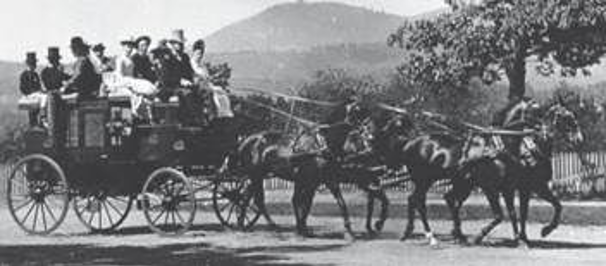
Humans are responsible for the concerns in today’s rideshare safety norms too. What’s a driverless car”? It is nothing but a computer on wheels. These onboard computers, radars, lidar, cameras, computer vision and host of other tech packed into the mobile computer uses life information, synched with a high performance cloud (internet) , having a super high bandwidth network, makes all the decisions,. Including that of safety. Even now, how is it our responsibility? To that my question who trained these onboard computers, servers in various locations and the intelligence that drives it? The whole eco system of self-driving cars runs on split second decision making using trillions of data points that are constantly being fed to the control unit. The onboard and its internet connected server computers use deep machine learning algorithms designed by man. Machine learning is when a computer is fed a particular habit to learn and a answer provided that this action or observation is good or bad, it can go deeper into false positives and how neural networks work, but that’s not our story. So, let's get back to why this brief deep dive into safety concerns. Machines are form and gender neutral, they don’t understand unless you teach them what’s right and what’s wrong and then they build on that what is taught, that’s the learning model of the car as well. The reason the young man died in the fatal car crash in his tesla is because the Tesla’s cameras “could not” sense that the white huge truck is an obstacle and it must slow down and halt but rather went and rammed into it killing its occupant. So also, the recent case of the pedestrian woman in Arizona who was killed by an UBER self-driving car, Was it the woman the car targeted, I would say “no”. My perspective along with that of many others is that the UBER self-driving car did not “see” the woman, and probably its “eyes” (the onboard sensors, cameras, etc.) failed to process the data in time to its onboard decision-making computer which controls the breaks to say, “hey there’s someone moving right in front, slowdown or halt”. In these cases, the developers who put those algorithms in place should rethink and probably redesign the way the decision making happens. It could be some serious cases in the future in terms of putting women and children at risks. The makers, I’d avoid pointing only to the developers, must consider looking at Aslimoar AI principles that we talked about earlier this paper. When we teach computer a logic and ask it to continue to learn on those grounds it's going to do so pretty fast and either for good or at the risk of disastrous collateral damages. It's our responsibility! Us as builders, us as developers, us as AI trainers building deep self-running tech and us as user or consumers of the services. Now let's look at rideshare. In the future of rideshare even owners ships and the entire living ecosystem will be at play to protect lives, safeguard our women and children. As our responsibility we’d have to look at not only the onboard decision-making computer in a self-driving car and how we program it to provide services, we’d also have to look at the companion apps and commands of operations. These functions and the eco system is designed to
learn, build use cases, authenticate and execute the learnings. MIT has this “Moral Machine” platform where you can be the judge and get to play a moral decision-making quiz towards
learn, build use cases, authenticate and execute the learnings. MIT has this “Moral Machine” platform where you can be the judge and get to play a moral decision-making quiz towards
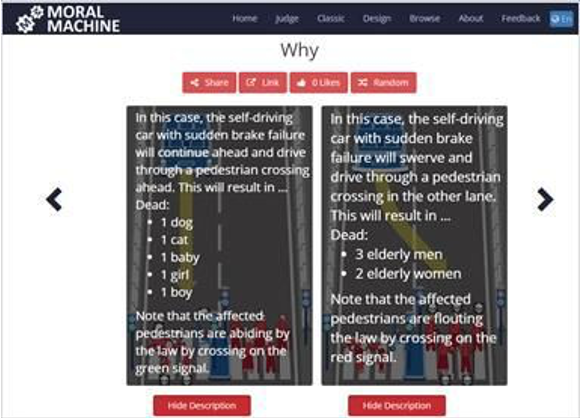
building decisions for an autonomous vehicle. Its scary to go through some of the scenarios about making decision on behalf of an autonomous car on whom to kill in an eventuality, for example in the even of failed brakes who should the self-driving car kill while heading towards pedestrians crossing? The machine is unbiased, for us to be safe and especially woman it is our responsibility to teach the intelligent machines what’s morally right or wrong. But that’s a long way ahead as artificial intelligence has not developed into super intelligence. Super intelligence is when a machine super seeds the intelligence of man. If precautions and right training is given to the advent of intelligent car design, it can draw on those safety norms in case of all scenarios and learn to protect rather than kill or destroy or even “drive away” overlooking a potential serious life-threatening situation. It is our responsibility to build hack safe systems that are onboard and as well as on the servers that communicate with the rideshare service. Building hack-safe systems are necessary as even if the whole car is safe it could be hacked to abduct the passengers and hold them for ransom or worse in case of intelligent sexual predators, intelligent pedophiles and systems hackers.
Women have to take precautions before they leave the house in order to feel protected and be safer. Why do we need to be aware of our clothes and our surroundings? We can’t wear headphones while walking at night because we won’t be able to hear anyone approaching from behind. Women have become so used to being aware and guarded we don’t even see it as a problem anymore. Is that the way we want to live? Being fearful of walking at night? Hiding our gender so we don’t get catcalled. Why should tech add to the woes of woman and children, its is our responsibility to build safer well protected systems.
The need for safety is to be addressed in solidification of mechanisms to approve and authorize the use of self-driving rideshare for the autonomous cabbie companies and the continued use of computational advancements to ensure safety. For example, the main tech behind rideshare and self-driving is based on data, machine learning, advanced imaging, spatial sciences, face and identity recognition, etc. this same background can be used to alert, predicate and advocate the right use of technology which is still in its very nascent stage. The environment for a rideshare in today’s world is colliding with the systems and laws that still favor traditional ways. If the safety had to be address, it’s at the grassroot of the tech, compliances and the ecosystem for rideshare, the coexistence of traditional and autonomous and how it can support our world to be safer.
Safety concerns are to be addressed at governance, laws, compliances, technology, engineering and above all the design of rideshare systems and all that falls into the gamut of the world of rideshare, with or without a driver. For women, there’s already some good initiatives like the women only drivers and rideshare service. In the case of Artificial intelligence most of them are more feminine, Example alexa, Siri etc. These robots are distinguished as female or male. The technology isn’t distinguishing itself it is due to the human influence. The designer, engineer or even the user is the one dictating how these robots are perceived. Technology that is adaptable and can-do things we as humans could never do is being categorized as women or men.
It is our responsibility as builders, makers, creators and users to come together and ensure
the systems designed today have a moral side to its Neuromorphic chips, which are more like brains making decisions that would take live driving data and process it morally towards the benefit and safety of humans, rideshare safety is one of its strong use cases. A morally right decision-making systems would have averted the death of the woman in Arizona, giving the ability to the car’s sensors to make right decision like a human would on a road.
the systems designed today have a moral side to its Neuromorphic chips, which are more like brains making decisions that would take live driving data and process it morally towards the benefit and safety of humans, rideshare safety is one of its strong use cases. A morally right decision-making systems would have averted the death of the woman in Arizona, giving the ability to the car’s sensors to make right decision like a human would on a road.